I’d like to introduce this guest blog by Adam Wray
Introduction
When I was preparing to deliver our Walkthrus CPD, I engaged in a discussion with Oliver Caviglioli for advice on what underlying theme could link our clusters of Walkthrus for our special school for high-functioning autistic children. He suggested using the cognitive science of cognitive load and dual coding theories, especially the transient information effect. This got me on a path to more deeply understanding cognitive load and dual coding theories, and how the different challenges of our SEN learners, and the strategies that we use to help them, could be illuminated by these theories. As I was digging deeper and wider into the associated cognitive science research when I came across Predictive Processing / Active Inference, (I will refer to these ideas as Predictive Processing in this text ). Predictive Processing is described as the latest thinking on how human cognition works, and potentially a “unifying theory” in cognitive science, neuroscience, psychology and the like. This theory appears to be no longer in the “cutting edge” of cognitive science but is now being established as a broadly applicable theory/model of how cognition/consciousness may work. Through Peter Vermeulen’s (@petervermulen) excellent book (Vermeulen, P., 2022,Autism and the predictive brain: absolute thinking in a relative world) and subsequently through Anil Seth and Andy Clark’s latest books (Clark, A., 2023. : The experience machine: How our minds predict and shape reality. Seth, A., 2021. Being you: A new science of consciousness ),
I found that the predictive mind theory provided a link between the symptoms of autistic people, the physical neuroscience, and the specialised strategies that we know work to help autistic people. Whilst very useful in the specialism of Teaching Autistic students, I have also concluded that the cognitive science of Predictive Processing can be very helpful in general education/pedagogy.
As with other cognitive science theories and models ( like working memory limits, dual coding theory, Cognitive load theory) I think that if teachers can gain an understanding of predictive processing it will positively influence their teaching, and further, I think it can really help in having empathy and understanding for our students, especially for those of our students who’s neurodiversity or social-emotional and mental health affects their learning.
Outline
The question is how does our mind construct reality? It has long been established that each of our senses of reality is a model created by our minds from the electrical and chemical signals coming to us via our senses. We don’t actually experience the light/sounds/smells, we interpret the signals from our sense organs to construct our own view of reality. This can be compared to interpreting the shadows on a net curtain and concluding a cat is sitting outside on a sunny day.

Traditional Model of Cognition

Predictive Processing model of Cognition – base
However, what predictive mind theory proposes is that it’s almost the opposite of this. the starting point is not processing the senses, but rather the generation of a prediction. It proposes that our mind uses prior knowledge and experience of the world from its long-term memory, and from working memory of the most recent past, and constructs a predicted model of what reality will be like next (or now).

This predicted model predicts down to the details what it should expect the senses should be if that prediction is accurate. Then it compares its prediction to what the senses are actually receiving from the world. If the senses match the prediction to a level of confidence, then all is good, and the mind keeps going with the path of its predictions. If the senses don’t match the prediction, then Prediction Errors are generated. These are then fed back into the prediction engine to correct future predictions.
These Prediction Errors are the feedback from comparing your prediction to your actual senses that allow you to adjust your predictions to be as close a representation of real-world as possible. So even though your perception of reality, your prediction, is effectively a hallucination, it is a “controlled hallucination” (Clark, A., 2023. ) that closely approximates the real world by virtue of being constantly corrected by the prediction errors.
There is good evidence to support the brain wiring supports this model as it has been observed that there are a much higher ratio of neurons going from the higher level brain regions towards the lower sensory organ regions than going the other way, in some areas the “downward” neurons outnumber the “upward” neurons by 4:1.(Vezoli, J,2021) This supports that the predictions being translated into lower levels have the highest bandwidth and the “prediction errors” need a much lower bandwidth as corrections going back up. The way errors are used to communicate change and minimise bandwidth is very similar to the techniques use in mpeg videos to compress the amount of information needed.
This organisation and structure of the brain is efficient and allows us to respond quickly to our environment as we can predict the most likely set of next events and can pre pre-empt them and prepare ourselves. For example, predicting we may need to take action and release adrenalin to prepare the body for rapid action, or moving and lining up our tennis racket to meet and return the fast serve.
Precision – Handling noise, driving attention
The reality of our world is that our sensory data signals are actually very noisy. We can think of all the other noise in a room compared to the specific conversation we are interested in following, All the different light signals coming into our eyes apart from the information we need to focus on, or even trying to follow the road on a rainy night or foggy day. If we are trying to interpret all the data we receive we would be generating lots and lots of errors that are not helping us modelling the real world.
The way the Predictive Processing system in our brains handles this is to have a kind of “Fuzzy Logic” when comparing our predicted sensory picture of the world to that from our actual senses. This is such that we can adjust the weighting of how much to believe our prediction Vs. the signals coming from our senses.

If I have high confidence in my prediction, (it has high precision) I set a very high threshold for generating errors. So this would mean if the sensory data roughly matches my prediction then I won’t generate any corrective errors and carry on allowing my future predictions to carry on the same theme. Only if my predicted sensory picture is way off would I generate prediction errors. If I am no so confident in my prediction (it has low precision), I want to put more weight on what my senses are telling me, then I will lower the threshold that errors get generated, so I get more prediction errors that can improve my future predictions.
By adjusting the confidence (known as precision in Predictive Processing), I can adjust and pay more attention to my senses when I need to and ignore the noisy signals when I need to.
Thus, the adjustment of precision of my predictions is the mechanism by which attention is managed.
Evidence the Brain Uses Predictive Processing
This sounds like a great theory – but is there evidence to show this is true?
Binocular Rivalry
One source of the is evidence is binocular rivalry. In binocular rivalry a separate image is shown to each eye and the observer is asked to describe their experience.
If possible, grab a red and a blue filter and put them on separate eyes, a pair of the colour 3D glasses. The effect can also be seen without the filters but to a lesser extent.

What typically happens after looking at the image for a little while is that one of the images will be very dominant, and the alternate image virtually disappears. This remains stable for a short time, then your view of the image transitions to the alternate image. This cycles backwards and forwards every few seconds or so.
What Predictive Processing proposes is that you only generate one version of your reality model – a prediction at once – the one you think is most probable at the time. You then compare what this prediction would generate in senses does a fuzzy compare. You have a reasonably high confidence in your prediction so when large parts of the senses match with your prediction, you stick with that prediction being the most likely. You do still generate prediction errors, but these are not at high enough a threshold to suggest a change in the prediction. However, after a while of sustained prediction errors the probability this is right prediction reduces, and so you switch to the alternate image to see if that is a better fit. The alternate image produces a high amount of confirmation from the senses, so you keep that prediction for a while before the sustained prediction errors force a change again.
If you were simply constructing a sense of reality from your senses, then you should always see a mixed view.
It is interesting to note that autistic people tend to see much faster oscillations – indicating their tendency to put more weight to sensory inputs than their predictions. On the other end of the scale, people with diagnosed schizophrenia have much longer oscillations, sometimes only fixing on one image showing how they can put more weight onto their predictions, ignoring strong sensory input to the contrary. The majority of “neurotypicals” fit in a distribution in the middle.
Reading Incomplete text
Try reading the jumbled-up text. Your brain is quickly able to interpret the mixed-up words. Predictive Processing proposes that you can do this because you are predicting the words coming next, and if they match to a reasonable level (because you have high confidence/ precision in your prediction), then you continue with your predictions on that theme.

Other examples will be used later to highlight the strong effect of Priors.
Handling Prediction Errors
Prediction Errors are a key feature in predictive processing. They communicate that our current prediction is not correct and the self-organising system of mind is designed to work to minimise the rate of prediction errors. So it is important to look at what we can do to handle the prediction errors such that future predictions have fewer errors by better matching the actual sensory data. This is especially important to us as educators as this is where learning happens.

No Error
A key element in predictive processing is that the presence of no – or rather errors below a threshold- means the current prediction scenario should be continued. This is the state our prediction processing system is designed to strive for. It means our predictions match what is happening in the real world outside our heads as closely as possible, whilst ignoring any noisy signals from our senses.
Adjust
We are getting rates of prediction errors that indicate our current prediction scenario is not quite right. We can adjust the scenario – it could mean things need to be faster, slower, a bit to the left, warmer etc. We are keeping within the main high-level scenario/narrative but adjusting some of its parameters. The changes in our future predictions should cause our prediction error rate to decrease as we approximate reality more accurately.
Alternate
Our prediction error rates are higher, or more sustained, and we need to make a bigger adjustment. We need to select a different high-level scenario about what is going on that may better fit what our senses are telling us. We switch to a different scenario/narrative for our future predictions with the aim that this will reduce future prediction errors.
Action
We take some kind of action which will better make our future sensory input match our predictions. The main proponents of predictive processing would argue this was the most important response, and where the predictive process system came from. The actions we take are generated by us sending nerve signals out down our motor neurons to our effectors, our muscles, our glands to make changes to the physical positioning of our body parts, or perhaps more importantly, changes to our metabolism, our internal systems for regulation. In cognitive/neuroscience there is significant work going on in exploring this idea of embodied cognition and how tightly bound our interoception and management of our homeostasis is related to our cognition and consciousness.
Learning in Predictive Processing
As educators the mechanisms of learning are of great interest to us. First I think its worth reviewing the purpose of learning within the predictive processing model. The whole point of the brain is to keep its organism alive. Predictive processing proposes that the architecture of the brain has developed to be a self-organising system which minimises prediction errors. This needs to happen “in the moment” but a successful organism will survive in the long term. Therefore, it will benefit from adaptations that work to minimise prediction errors in the long term. In the predictive processing model, one such adaption is the long-term memory such that the schema and narratives that have helped shape successful predictions can be stored for future use, and recalled to help make future successful predictions that minimise long-term prediction errors.
The well-referenced quote, “learning, in turn, is defined by a change in long-term memory” ( Kirshner, Sweller & Clark, 2006) is a good lens through which to examine how predictive mind theory relates to learning.
In the prediction processing model, long-term memory is updated the signal that our schema and narratives stored in our long-term memory need updating is a higher level of prediction errors. We update the priors stored in our long-term memory so that we are better at predicting in the future – we are minimising long term prediction error rate.
If priors we retrieve from our long-term memory make good predictions, then we have low rates of prediction error. You could argue we are in a flow state, we are performing well, in our sport, our music, in our final exam, or in our day-to-day tasks. In this state, although we may be getting some re-enforcement of our long-term memories, keeping them salient and remembered, we are not making changes to our long-term memory, so we are not learning we are performing well.

So at very low rates of prediction error we are performing.
As the rate of prediction errors increases, we start to update the priors ( schema and Narratives) stored in long term. We are now learning. As the rate of prediction errors increases, we make bigger and more frequent changes in our long-term memory, we learn faster/more productively.
However, as we increase the rate of prediction errors, we start to hit the limits of the bandwidth of our prediction error circuitry. This can be from large errors in a specific sensory area or multiple sets of errors across our sensory landscape. The quality of the information we feed back up to our higher-level brain areas starts to decline, and our predictions become less precise, further loading the prediction error pathway. Unless we can find an alternate set of predictions that will better match our sensory input, we are in a state of stress and confusion and can easily move into an overload state. As the prediction error pathway’s bandwidth gets filled, we are sensing danger, and we generate anxiety and fear emotions which start preparing our bodies for fear responses. We might freeze or disconnect, we may flee the situation, we may invoke our aggression and be ready to argue and fight. Our brains try to find a route to get our prediction processing system back into control and within the bandwidth limits of the prediction error pathway again.
In the extreme, this becomes the dysregulated state we see in special schools more frequently.
Note that the ranges of prediction error rate % for each state are rough estimates, provided to illustrate the ideas, the boundaries are blurred and will vary from person to person and in different contexts. These are not based on any specific research evidence, apart from the observations that a 70-80% pass rate seems optimal for productive learning.
The Importance of Priors
The role of priors is critical in the prediction processing model, and especially important for us to consider as educators

Priors are what are used to generate predictions. These are of the following main forms:
- Episodic memory(narrative) of what has just been happening.
- Narrative and schema retrieved from long-term memory relevant to the current situation.
The priors held in long-term memory can be:
- Schema/models of how things are in the world
- Faces point out
- Light shines down on objects
- Shadows make things look darker than they are
- Cats have 4 legs are furry and meow
- Phonemes that match a word
- …etc.
- Narratives / episodic memories
- Typical sequences of events
- Routes
- Methods
- Stories
- grammar of sentences
We can think of our prediction engine and priors as a classic complete-the-sequence process. You cannot make a prediction without the prior terms. The better or more comprehensive your set of priors, the better your predictions. You can also make better predictions by referring to your memory of similar sequences.

As we experience a situation, we recall priors based on the episodic memory of what is just happening to help us make the next prediction—to shape the prediction scenario.
We must remember that we are not discussing a sequence in a math question, but rather, we are unconsciously processing these predictive sequences for everything we experience: what the next phoneme in speech will be; what the next grapheme in reading is; where the shape that forms the cat’s head will appear next in my visual field.
Our priors shape the prediction – one set of priors could generate an accurate prediction, and another set of priors could generate an inaccurate prediction.
To demonstrate the power of priors and experience predictive processing in action, listen to the following clip of sound wave speech. This is a sound file of speech where most of the frequencies have been removed. You will then be played the full speech and then listen to the original file again.
Once the prior of the actual speech has been established, your actual experience is to hear and understand the sentence that you previously could not comprehend. Initially, your priors did not allow you to predict the sounds to make understandable language. However, once you had been given the prior of the full sounds, you were then able to use the priors to predict the words, and when you compared your predictions to the scant sensory input, it matched sufficiently that you maintained your predictions as your sense of reality.
Now an example showing how our inbuilt prior knowledge of how the world works influences our visual experience.
Square A appears to be a “white” square, and square B appears to be a “black square.

However, Squares A and B are actually exactly the same shade of grey.

Our strong prior about how shadows effect the relative luminosityof objects and our prior experience of checkered patterns is driving our perception. It is causing an unconscious high precision on our predictions and ignoring actual sensory data on the brightness of light coming from different regions of the board. The context of the situation is key to the interpretation of what the sensory signals are communicating.
In this sense our perception of the shadow on the chessboard is completely our fabrication / controlled hallucination of what is happening in the real world outside our heads. It is worth noting that no matter how much you try to consciously try to see the two squares as the same shade you cannot. The inbuilt prior about how the world works is too valuable to long-term prediction error reduction be consciously overturned.
The sinewave speech demonstration shows the effect of the “just happened” part of the priors and the chessboard shows the effect of strong schema of how the world world works.

The critical factor for us as educators here is the power and importance of priors. We need to be aware that our students will have their own set of both the “just happened” priors and their own unique set of priors stored in their long-term memory. These long-term memory stores will have both the schema that relates to how the world works ( like the effect of shadows) and their own unique set of narratives and schema from their own experiences. When they are sat in front of you in the classroom they will each have this unique set of a prior stream, from which they will be predicting what is going to happen next/now. when observing/listening to the same content, there will be a variation in how well their predictions match the next parts of the lesson. Different students will actually perceive your lesson content differently, and they will tune in their predictions at differing rates.
Educational discourse is full of advice for teachers to take great care not to assume what students are learning from their lessons. You have to be very wary of assuming students have a certain level of knowledge of general vocabulary and how things work in the world. You, as the teacher, have the curse of the expert, and it’s hard for you to think like the novices you have in front of you.
Teachers as Prediction Error Managers
As educators, we seek to optimise learning and are motivated to manage prediction error rates within the optimum range.
With an awareness of the mechanics of predictive processing, let’s take a look at how we might become Prediction Error Managers to optimise the learning of our students.
Within this blog post, I will cover an overview of each of these areas and intend in future blogs to delve more deeply into how the lens of predictive processing can be helpful to us as educators. How it might provide an underlying cognitive science backbone to different areas of good practice.
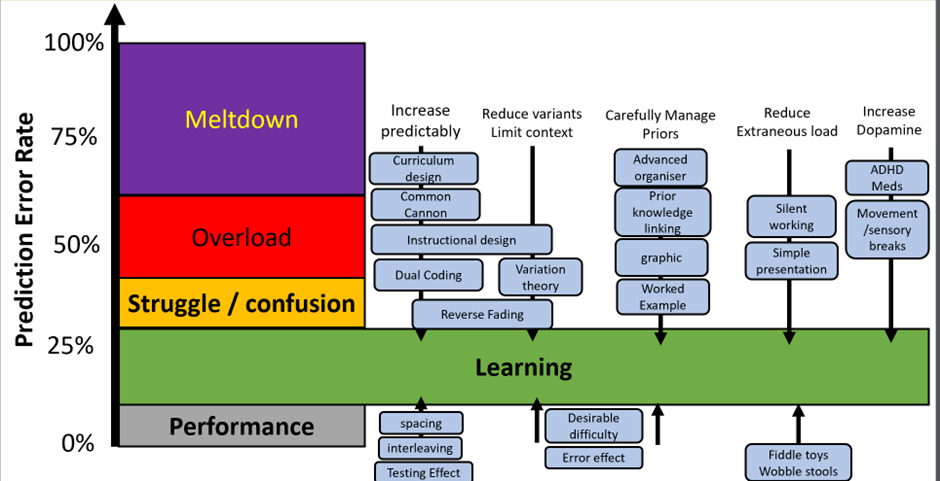
Much of the educational discourse informed by cognitive science to date has been about the limitations of working memory and how we are often overloading our students. Within the predictive processing model, we therefore want to look at how we can reduce the rates of prediction error to get our students into the range for optimum learning. We will also take a look at the opposite and think through ways in which we can increase students’ prediction error rates to move them up into the optimum learning range.
The first area to look at is predictability and variation. If our students are being overloaded, we can aid them by increasing the predictability and reducing the variability in their lesson content, and in the physical and social environment. We can think of this in terms of cognitive load theory. The types of things we can do are to have routines, set formats for worksheets and questions, common language, clear logically progressed instructional sequences. We can check for understanding before we introduce new materials. The key in all of these techniques is to be very aware of only introduction variation/new elements in the areas you want your students to learn. If you are introducing a new means of participation or routine, then the content that is being worked with should be familiar. Variation theory, as described by Craig Barton, is a good example. Good curriculum design carefully considers logical sequences to ensure component knowledge is covered, and new material is introduced at a rate which allows students to progress.
The ideas in Asubel’s meaningful learning and explicit instruction come into play here. We want to carefully share the visual schema of how information in a topic is related and explicitly communicate any links or places in a structure. Utilising dual-coded diagrams and graphic organisers helps limit the variation to where the new knowledge fits into the whole.
The use of worked examples and reverse fading scaffolding, including I do, we do, you do are all designed to increase predictability and limit variation initially, and then gradually decrease the support, decreasing the predictability and increasing the variation as the student’s priors (schema and narratives) become more solid.
When we think about the low learning effect when prediction errors are low, we will seek ways to increase the level of prediction errors. Predictive Processing helps us understand why re-reading, writing out notes and blocked practice have low productivity in learning. It helps us understand why students benefit from more highly scaffolded questions when their knowledge in an area is new or weaker, and why the gradual increase in difficulty/reduction in scaffolding and movement to more open questions is helpful. @sarahCottingham in her recent podcast with Ollie Lovell on the @EducationReadingRoom podcast was interested in exploring why familiarity “tricks us” into not learning. I think predictive processing clarifies these reasons; there are insufficient prediction errors to generate updates of priors in long-term memory. We need to up the desirable difficulty, the germaine load, and the task complexity – reducing the predictability and generating an adequate level of prediction errors to stimulate productive learning.
It also helps understand the underlying cognitive science behind the testing effect, spaced learning, interleaving, the error effect and others as these are creating prediction error events.
Cognitive Load theory introduces us to the idea that “extraneous load” starts to consume our limited working memory. Extraneous load is anything outside the focus of our learning. When we look through a predictive processing lens, we can see the extraneous load as an additional sensory stimulus that the brain will work to provide a prediction with which to explain it. We could consider extraneous load a parallel part of the sensory field that consumes prediction and prediction error bandwidth. However, we need to note the learning from observing the binocular rivalry experiments which indicate strongly that our unconscious mind selects a single prediction scenario to test against its senses. In this case, distractions/extraneous load may completely take over all our bandwidth (at least periodically), meaning the focus of our learning is ignored and information lost. I think this explains our visceral experience in our classrooms with chatter and distractions. It is absolutely highlighted for me in an autistic school where rain falling on the skylights, or inconsistent fonts can completely take students from their learning. In this sense, the impact of the extraneous load is not a continuous variable, but binary, so management of extraneous load and distractions becomes highly influential in managing students’ prediction error rates into the optimal learning zone.
Neurodiversity, Anxiety and SEMH
Everyone’s brains are wired differently, but they have many common elements. Consequently, we will see variations in the levels of prediction errors different people can manage and continue to learn and differing levels at which people are successful at updating or retrieving priors from long-term memory. We see this in the classroom as variations of student’s rate of learning.
For neurodiverse people (Autistic / ADHD), their different brain wiring means that they put significantly more weight on their sensory input, trying to make sense of all the noisy sensory signals, and as a consequence, have inherently higher levels of prediction errors. We know from our work in special schools that these students benefit from extra focus on minimising change and variation, keeping the environment as predictable as possible. This then may allow them to be in the “learning” zone. But it is worth noting that most of the time, these students are in an anxious/ stressed state due to their high levels of unconscious prediction errors.
Note students with anxiety and other SEMH challenges have reinforcing patterns established in their priors which cause them to misinterpret sensory signals and maintain a belief that they should be in a fear response. They are also in a similar state of anxiety and stress, and it makes it harder for them to be in the optimum learning zone.

Managing Prediction Errors by shaping Priors
Understanding the power of priors to shape experience and learning can help us as educators as we are able to shape the priors of our students. We can aim to get as much commonality in the key parts of the priors to allow our classes to progress with their learning. Some of the ways in which we can do this are:
- Carefully Structured Curriculum.
- A common cannon of texts – don’t assume students’ prior knowledge.
- Advance organisers( as per Asubel).
- Linking to, and checking required prior knowledge.
- Giving concrete examples that are within all students’ experience.
- Starters that settle and are related content of the lesson ( in structure or content)
- Carefully thought through and scripted Explicit/Direct Instruction/explanations.
- Use of graphic organisers / dual-coded diagrams.
- Regular Checks for listening and understanding.
Conclusion
I hope you have found the explanation of predictive processing as interesting, thought provoking and useful as I have. I really believe this latest thinking in cognitive science can be as helpful and insightful as previous cognitive science ideas have been for educators. In this article, and in the development of these ideas, I believe we are just the very starting point of how we can apply them into the field of education. I plan to explore these ideas in future blogs, and I strongly welcome contributions and ideas from us all.
The folks at @cogscisci have been very supportive and have setup a channel on their excellent Discord server so we can continue the discussion and development of these ideas.
The link to join if you have not already is here: https://discord.gg/vYwvXE6Bjr
I will be presenting these ideas at at ResearchEd SouthWest, Gloucester on 27th April 2024 https://researched.org.uk/event/red-south-west/
and at the @cogscisci conference 2024 – St Albans on 31st May 2024. https://cogscisci.wordpress.com/2024/02/08/cogscisci-st-albans-2024/
I can be found on Twitter,X and Threads as @AdamnWTeach.
References / Supporting Evidence
I outline here some of the major players and sources of information I have used whilst trying to understand this area of cognitive science. This is not an exhaustive list, and I have no doubt left out some valuable contributions to the field. However, I present them here so that others can explore further
Main players / sources
Karl Friston, Prof. Neuroscience at UCL, is perhaps the most central figure in the development of predictive processing. Friston’s work on the “free energy principle” posits that biological systems, like the brain, strive to minimise the difference between predicted and actual sensory inputs. His theoretical contributions provide a unifying framework based on physics concepts, that has been widely influential in neuroscience and cognitive science.
Parr, T., Pezzulo, G. and Friston, K.J., 2022. Active inference: the free energy principle in mind, brain, and behavior. MIT Press.
https://youtu.be/NwzuibY5kUs28 May 2020 |
Andy Clark, Prof. Cognitive Philosophy University of Sussex, has been a major influence in this field, broadening the scope into how many aspects of cognition and consciousness can be supported with the predictive processing model. He is also strongly developing the philosophical aspects of embedded cognition.
Clark, A., 2015. Surfing uncertainty: Prediction, action, and the embodied mind. Oxford University Press.
Anil Seth, Cognitive & Computational Neuroscience University of Sussex, has also been shaping the debate on predictive processing. His focus has been on understanding consciousness, and integrating neurology and imaging, through to how computational models can be developed to demonstrate and use the related theories.
Seth, A., 2021. Being you: A new science of consciousness. Penguin.
Royal Faraday Lecture: – 26th March 2024 https://youtu.be/ghxy9YTm5-s
Chris Frith, Emeritus Professor of Neuropsychology University College London, has developed predictive processing in the area of social cognition and relationship with others, as well as using it to understand mental illness including schizophrenia.
Uta Frith, Emeritus Professor of Cognitive Development University College London, have been key in exploring how predictive processing relates to social Cognition, and in relation to autism and dyslexia.
Frith, C. and Frith, U., 2023. What Makes Us Social?. MIT Press.
Frith, U., Frith, A. and Frith, C., 2022. Two Heads: Where Two Neuroscientists Explore how Our Brains Work with Other Brains. Bloomsbury Publishing.
Jakob Hohwy, Prof. of Philosophy, Monash University, has developed the field of predictive processing, providing thinking on how the how predictive processing can account for the phenomenological aspects of conscious experience, and has done much work on producing empirical testing and evidence to support the theories.
Hohwy, J., 2013. The predictive mind. OUP Oxford.
Lisa Barret Feldman, Prof of Psychology North Eastern University, has contributed by applying ideas of predictive processing in the area of emotional cognition.
Barrett, L.F., 2017. How emotions are made: The secret life of the brain. Pan Macmillan
Peter Vermeulen, Educationalist Autism in Context, has done an excellent job in interpreting predictive processing, and expanding on how it can be applied to understanding neurodivergent, particularly autistic people.
Vermeulen, P., 2022. Autism and the predictive brain: absolute thinking in a relative world. Routledge.
Selected Research Papers on predictive processing
Clark A. Whatever next? Predictive brains, situated agents, and the future of cognitive science. Behav Brain Sci. 2013 Jun;36(3):181-204. doi: 10.1017/S0140525X12000477. Epub 2013 May 10. PMID: 23663408.
Friston, K. The free-energy principle: a unified brain theory?. Nat Rev Neurosci 11, 127–138 (2010). https://doi.org/10.1038/nrn2787
Clark A. Whatever next? Predictive brains, situated agents, and the future of cognitive science. Behavioral and Brain Sciences. 2013;36(3):181-204. doi:10.1017/S0140525X12000477
Seth, A. K., & Hohwy, J. (2021). Predictive processing as an empirical theory for consciousness science. Cognitive Neuroscience, 12(2), 89–90. https://doi.org/10.1080/17588928.2020.1838467
Vezoli, J., Magrou, L., Goebel, R., Wang, X.J., Knoblauch, K., Vinck, M. and Kennedy, H., 2021. Cortical hierarchy, dual counterstream architecture and the importance of top-down generative networks. Neuroimage, 225, p.117479.
Edelson, Edward H. (2005). “Checkershadow Illusion”. Perceptual Science Group. MIT. Retrieved 2007-04-21.
PAPERS ON PREDICT PROCESSING IN EDUCATION
Sanchez, S. (2021). Embodied Learning: Capitalizing on Predictive Processing. In: Arai, K. (eds) Intelligent Computing. Lecture Notes in Networks and Systems, vol 285. Springer, Cham. https://doi.org/10.1007/978-3-030-80129-8_34
Köster, M., Kayhan, E., Langeloh, M., & Hoehl, S. (2020). Making Sense of the World: Infant Learning From a Predictive Processing Perspective. Perspectives on Psychological Science, 15(3), 562-571. https://doi.org/10.1177/1745691619895071
May, C. J., Wittingslow, R., & Blandhol, M. (2022). Provoking thought: A predictive processing account of critical thinking and the effects of education. Educational Philosophy and Theory, 54(14), 2458–2468. https://doi.org/10.1080/00131857.2021.2006056
EDUCATION RELATED REFERENCES
Cottingham S., 2023, Ausubel’s Meaningful Learning in Action. John Catt
Kirschner, P.A., Sweller, J. and Clark, R.E., 2006. Why minimal guidance during instruction does not work: An analysis of the failure of constructivist, discovery, problem-based, experiential, and inquiry-based teaching. Educational psychologist, 41(2), pp.75-86.
Thanks for writing this Adam.
Having seen you explain it live at ResearchEDSouthWest yesterday, I had to come straight back to it today to recap. If really is quite a comprehensive synthesis of a wide range of ideas, tied in to some practical take-aways for teachers.
Marvellous.
LikeLiked by 2 people
i’f like details about how to attend conference even if not speaking/
d.e.kornbrot@herts.ac.uk
LikeLike